Using Deep Learning to Classify Post-COVID-19 Lung Progression Phenotypes
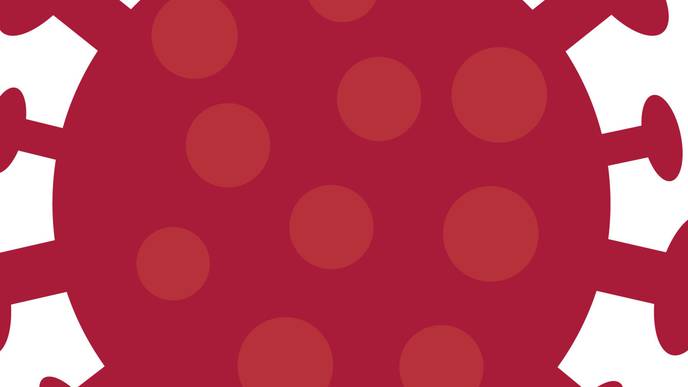
10/06/2023
Individuals diagnosed with different subtypes of long COVID or Post-Acute Sequelae of COVID-19 experience long-term physical, cognitive and mental health symptoms. As a result of the COVID-19 pandemic, researchers have begun to track the long-term health of COVID-19 survivors who recovered from the severe acute respiratory syndrome coronavirus 2 (SARS-CoV-2) infection.
To accurately diagnose and treat long COVID and its subtypes efficiently and effectively, Dr. Tianbao Yang, associate professor in the Department of Computer Science and Engineering at Texas A&M University, in partnership with the University of Iowa, has received a $3.7 million grant from the National Institutes of Health to develop self-supervised deep learning technologies capable of recognizing subtypes of post-COVID lung progression phenotypes.
According to Yang, lung progression phenotypes are different patterns or stages of disease progression and manifestation in the lungs. These phenotypes help categorize and understand the course of lung diseases such as chronic obstructive pulmonary disease, asthma, idiopathic pulmonary fibrosis and others. Understanding these phenotypes is pertinent when determining appropriate care measures for long COVID patients.
“Past epidemics caused by similar viruses, such as severe acute respiratory syndrome, show that the aftermath can last more than a decade,” said Yang. “Since the lung is the most common site of SARS-CoV-2 infection, the ability to evaluate effectively and comprehensively pulmonary sequelae in COVID survivors is critical.”
By using deep learning technologies, Yang’s research aims to understand the symptoms associated with impaired lung function in patients with post-COVID-19 diagnoses. Understanding the differences in symptoms and biomarkers is critical to making the correct subtype diagnosis and ensuring patients receive the proper treatment.
The biggest hurdle to developing this technology is the lack of data available since COVID-19 is still a new and emerging disease. Generally, deep learning technologies rely on a costly system requiring human-labeled data — physicians annotate medical images, which can then be used for medical image analysis.
To combat this problem, Yang is creating a contrastive self-supervised deep learning model that utilizes X-rays and CT scans to differentiate post-COVID-19 subjects from healthy subjects while simultaneously identifying post-COVID-19 subtypes. In other words, his technology can use artificial intelligence to pull information from unlabeled retrospective data. Using patients’ unlabeled images drastically increases the data available to train the model to diagnose and understand disease progression more accurately.
“We will develop advanced contrastive self-supervised learning to learn encoder networks from large-scale unlabeled X-ray and CT images,” said Yang. “This will enable us to develop an accurate classifier to effectively detect post-COVID-19 subjects from limited labeled data collected from COVID-19 patients.”
In addition, by analyzing the relationships between clinical and imaging biomarkers in different post-COVID-19 subtypes, the researchers will gain a deeper understanding of the underlying mechanisms behind the various forms of infection and their implications.
“Our long-term goal is to develop an integrated deep learning model that can assess lung images to assist with managing and treating long-term sequelae of post-COVID-19 subjects,” said Yang.
Moving forward, the researchers will conduct a longitudinal human subject study that tracks post-COVID-19 individuals 48 to 60 months after their initial visits to continue building data and developing the deep learning technology.
This research is a collaboration between Texas A&M, the University of Iowa Hospitals and Clinics and the College of Engineering at the University of Iowa.
Facebook Comments